Portland
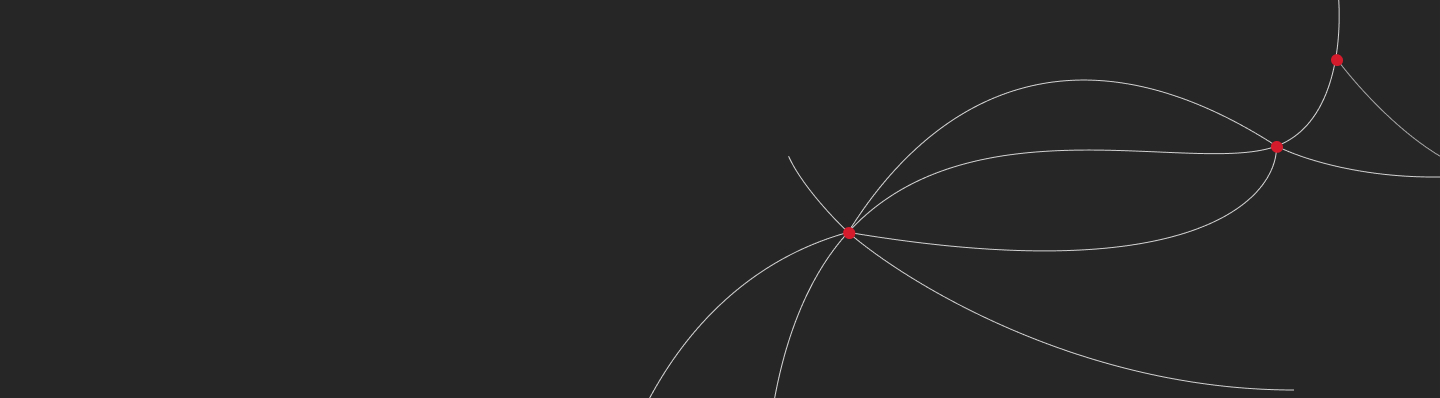
Master of Science in Data Science - Portland
Program Overview
An extensive core curriculum—designed jointly by Khoury College of Computer Sciences and the College of Engineering faculty—enables you to develop depth in computational modeling, data collection and integration, data storage and retrieval, data processing, modeling and analytics, and visualization. Electives from Khoury College of Computer Sciences, the College of Engineering, or a Northeastern partner college provide an opportunity to explore key contextual areas or more complex technical applications.
For the fourth year in a row, Glassdoor named data scientist as the best job in America. And as Daniel Gutierrez, managing editor of insideBIGDATA, told Forbes, "The word on the street is there's definitely a shortage of people who can do data science." Given the job prospects and shortage of talent, now is the time to advance your career in the data science field.
All Roux Institute programs provide content relevant to the urgent and emerging needs of industry in Maine and the rapidly evolving regional, national, and global economy. Opportunities for experiential learning will be concentrated in Portland, the state of Maine, and the Northeast region. Students are encouraged to pursue co-ops and special virtual Experiential Network projects with the institute’s founding corporate partners, a group of leading employers in Maine.
-
-
Full-Time
Part-TimeCommitment
-
2-3 years
Duration of Program
Unique Features
- This is an interdisciplinary program between Northeastern’s Khoury College of Computer Sciences and the College of Engineering.
- Courses are tailored toward technically or mathematically trained students.
Program Objectives
- Collect data from numerous sources (databases, files, XML, JSON, CSV, and Web APIs) and integrate them into a form in which the data is fit for analysis.
- Use R and Python to explore data, produce summary statistics, and perform statistical analyses; use standard data mining and machine-learning models for effective analysis.
- Select, plan, and implement storage, search, and retrieval components of large-scale structure and unstructured repositories.
- Retrieve data for analysis, which requires knowledge of standard retrieval mechanisms such as SQL and XPath, but also retrieval of unstructured information such as text, image, and a variety of alternate formats.
- Manage, process, analyze, and visualize data at scale. This outcome allows students to handle data where the conventional information technology fails.
- Match the methodological principles and limitations of machine learning and data mining methods to specific applied problems and communicate the applicability and the advantages/disadvantages of the methods in the specific problem to nondata experts.
- Carry out the full data analysis workflow, including unsupervised class discovery, supervised class comparison, and supervised class prediction; summarize, interpret, and communicate the analysis of results.
- Organize visualization of data for analysis, understanding, and communication; choose the appropriate visualization method for a given data type using effective design and human perception principles.
- Develop methods for modeling, analyzing, and reasoning about data arising in one or more application domains such as social science, health informatics, web and social media, climate informatics, urban informatics, geographical information systems, business analytics, bioinformatics, complex networks, public health, and game design.
Career Outlook
Northeastern's MSDS graduates have found success in a wide range of industries and positions:
- Data science analyst at MFS
- Fixed income quantitative researcher at State Street Corporation
- Data analyst at TJX
- Data analyst at Stop & Shop
- OCR imaging/natural language processing engineer at iQuartic
- Machine learning/OPS engineer at New York Life
Scholarships and aid
$55.4K
Tuition
Estimated Total Tuition
This is an estimate based on the tuition rates for Academic Year 2022-2023 and does not include any fees or other expenses. Some courses and labs have tuition rates that may increase or decrease total tuition. Tuition and fees are subject to revision by the president and Board of Trustees at any time. For more detailed information, please visit Student Financial Services.
Generous scholarships
The Roux Institute is currently offering generous scholarships to meet the financial needs of all students through its Alfond Scholars Initiative. Each award is determined by an individual assessment. And Northeastern alumni receive a Double Husky Scholarship —a tuition discount of 25 percent.
Learn more about the Alfond Scholars InitiativeCorporate tuition benefits
Many employers subsidize education for their employees. Speak with yours about any tuition benefits your company may offer.
Special military scholarships
For military veterans and servicemembers, a limited number of donor-funded scholarships are available even after all other aid has been awarded to help with commuting costs, childcare, and other costs of living.
Learn more about military scholarshipsFederal aid
You can apply for federal aid grants and loans through the Free Application for Federal Student Aid, or FAFSA.
Learn about the FAFSAAdmission Requirements
- Online application and fee
- Unofficial undergraduate/graduate transcripts (you can submit official transcripts from all colleges/universities attended at the time of admission)
- Statement of purpose that should include career goals and expected outcomes and benefits from the program
- Recent professional resumé listing detailed position responsibilities
- Three confidential letters of recommendation
- Unofficial TOEFL or IELTS examination scores (international students only)
Prerequisite Courses
The Master of Science in Data Science curriculum is tailored toward technically or mathematically trained students. To ensure that all students have the foundation necessary to be successful in this program, each incoming student must either complete two introductory courses at Northeastern or complete two placement exams administered one week prior to the beginning of the semester. The two exams cover fundamentals of computer science and programming skills and basic statistics, probability, and linear algebra. This admission requirement can also be fulfilled by successful completion of Introduction to Programming for Data Science (DS 5010) and Introduction to Linear Algebra and Probability for Data Science (DS 5020). The introductory courses are not counted as credit toward the degree but are included in the student’s cumulative grade-point average.
Admission Dates
Our admissions process operates on a rolling basis; however, we do recommend following the application guidelines below to ensure you can begin during your desired start term.
Please note this program will begin at the Portland campus starting spring 2022.
- International applicants: May 6
- Domestic applicants: August 1
- International applicants: September 15
- Domestic applicants: December 1
Program Curriculum
Experiential Learning
Co-op makes the Northeastern graduate education richer and more meaningful. It provides master’s students with up to 12 months of professional experience that helps them develop the knowledge, awareness, perspective, and confidence to develop rich careers. In addition to the esteemed faculty, many students enroll in the master’s programs largely because of the successful co-op program.
Graduate students typically have an experiential work opportunity following their second semester. This could be a six- to eight-month co-op or a three- to four-month summer internship. Those who initially experience co-op may have the opportunity to seek an internship for the following summer, or vice versa.
Student participation in experiential education provides enhanced:
- Learning, technical expertise, and occupational knowledge
- Confidence, maturity, and self-knowledge
- Job-seeking and job-success skills
- Networking opportunities within your desired career path
Northeastern’s co-op program is based on a unique educational strategy that recognizes that classroom learning only provides some of the skills students will need to succeed in their professional lives. Our administration, faculty, and staff are dedicated to the university’s mission to “educate students for a life of fulfillment and accomplishment.” Co-op is closely integrated with our course curriculum and our advising system. The team of graduate co-op faculty within the Khoury College of Computer Sciences provides support for students in preparing for and succeeding in their co-ops.
These multiple connections make co-op at Northeastern an avenue to intellectual and personal growth: adding depth to classroom studies, providing exposure to career paths and opportunities, and developing in students a deeper understanding that leads to success in today’s world.